05 February 2025
Our complex and dynamic mining value chains face ever-increasing challenges. The decisions we make today will impact us for decades to come. Learn how BHP is working to help future-proof strategic decision-making using a ‘digital twin’ approach combined with GenAI technologies.
Leveraging the latest tools and technologies to seek to quantify the impact of our strategic decision-making is essential to meet the growing demand for commodities critical to global trends such as the world’s growing and urbanising population and the global energy transition.
This article explores how the use of ‘digital twins’ combined with Generative Artificial Intelligence (GenAI) technologies is better enabling us to make the best strategic decisions possible.
Generative AI, can be thought of as the next generation of artificial intelligence. It's a form of AI that can create something new by learning from vast amounts of data. Unlike traditional AI, which follows fixed rules to analyse and process information, GenAI can generate insights, predict outcomes and suggest solutions – making decision-making faster, smarter and more adaptive.
Digital twins of our mining value chains
A digital twin of a mining value chain is a virtual replica of the entire operation, from the mine to the port. Through this virtual world, we are able to test different scenarios and see their impact before making real-world changes. This helps us make smarter, data-driven decisions while reducing risks and improving efficiency.
Example of the continuous data exchange between a physical asset and the digital twin.
Mining operations are highly complex, often involving multiple mines, hubs, railways, ports, conveyors, loaders and trains - all working together. With so many moving parts, it’s impossible for humans alone to optimise every decision. By combining digital twins with GenAI, we can process vast amounts of data to make better decisions to improve our productivity, reduce our costs and environmental impact, and make our operations safer.
Maximising future production outcomes
When we know what combinations of variables are required to deliver our production targets, we can focus our efforts on these things that matter most. Previously this was not always the case, as it can be difficult to quantify the production uplift from an improvement initiative, especially when there are many interdependent initiatives underway.
Through a digital twin and GenAI we can analyse our extensive historical data and current mine plans and operating context to predict realistic production outcomes. If these predictions identify production risks, we can take swift action to mitigate them. For example, a digital twin can identify the location of a bottleneck and help us decide how to address the root causes. Testing ‘what-if’ scenarios within the digital twin saves time and cost and is safer as we require fewer on-site trials.
We are using digital twins at BMA, Copper South Australia and Escondida to predict future production outcomes and identify key performance drivers, risks and opportunities. Through these digital twins we have found new opportunities including to optimise mine haulage, address ore fragmentation and material handling challenges, and debottleneck surface operations.
Empowering decision making at the front line
Our Integrated Remote Operations Centre operators must make quick decisions, but our operations are complex, and it is not always easy to determine the best decision at any given moment. For example, where should we send the truck? How many loading units should we operate? How should the dump be configured?
The BMA Autonomous Haulage Excellence Program has developed proprietary tools and in-house capabilities that uses AI, advanced analytics and digital twin modelling to predict operational performance. This allows us to act early before a problem arises. It is defining new ways to optimise and maintain our operations.
Although autonomous sites are highly complex and dynamic with lots of moving parts, they also present great opportunities to collect and use the vast amounts of data and information that was not available in the past. We can now supply tens of millions of data points to a machine learning model that does the heavy lifting with identifying the key drivers correlated with good performance and then mapping over time to predict variability across the mining system, as we flex operating conditions. This allows us to maximise performance by optimising our equipment strategies and driving rapid improvement back into the system. In a very recent example, it facilitated an increase in productive movement by 10% per annum at one of BMA’s autonomous operations.
Improving mine planning using probabilistic methods
Traditionally, our mine planners have used deterministic methods to create mine plans, where the output is fully determined by the inputs. But the world is full of variability, and we need to plan for this variability if we are to stabilise our mine plans and successfully execute them. We make thousands of decisions daily in operations. Each decision we make either increases or decreases the variability in the system. Which decisions are good decisions, and which are bad decisions? How do we choose? What are the impacts? How do we manage the impact of variability?
A key BHP strategic ambition is to transform mine planning to create more robust, stable and achievable mine plans. By incorporating probabilistic methods and ranges into mine planning we can better understand the impacts of value chain variability. To support this transformation, we are leveraging our digital twin and GenAI to test and mitigate the impact of variability on our operations. Our digital twin at BMA has uplifted decision-making confidence across the business.
Satisfying future global copper demand, despite supply challenges
We expect future global copper demand will grow, with looming global copper supply challenges. To increase copper production at Escondida, we use advanced analytics to understand the impacts of different ore characteristics and granulometry on semi-autogenous grinding (SAG) mill performance. A digital twin of the Escondida value chain and GenAI models inform ore blasting and blending strategies, identify mine areas with challenging ore characteristics, and support the implementation of SAG mill model predictive control. By mitigating the impacts of varying mineral characteristics, we have reduced monthly production losses due to granulometry by an average 70 per cent.
We are providing the same data-driven informed decision making across the business to support copper production, including at Copper South Australia and Spence.
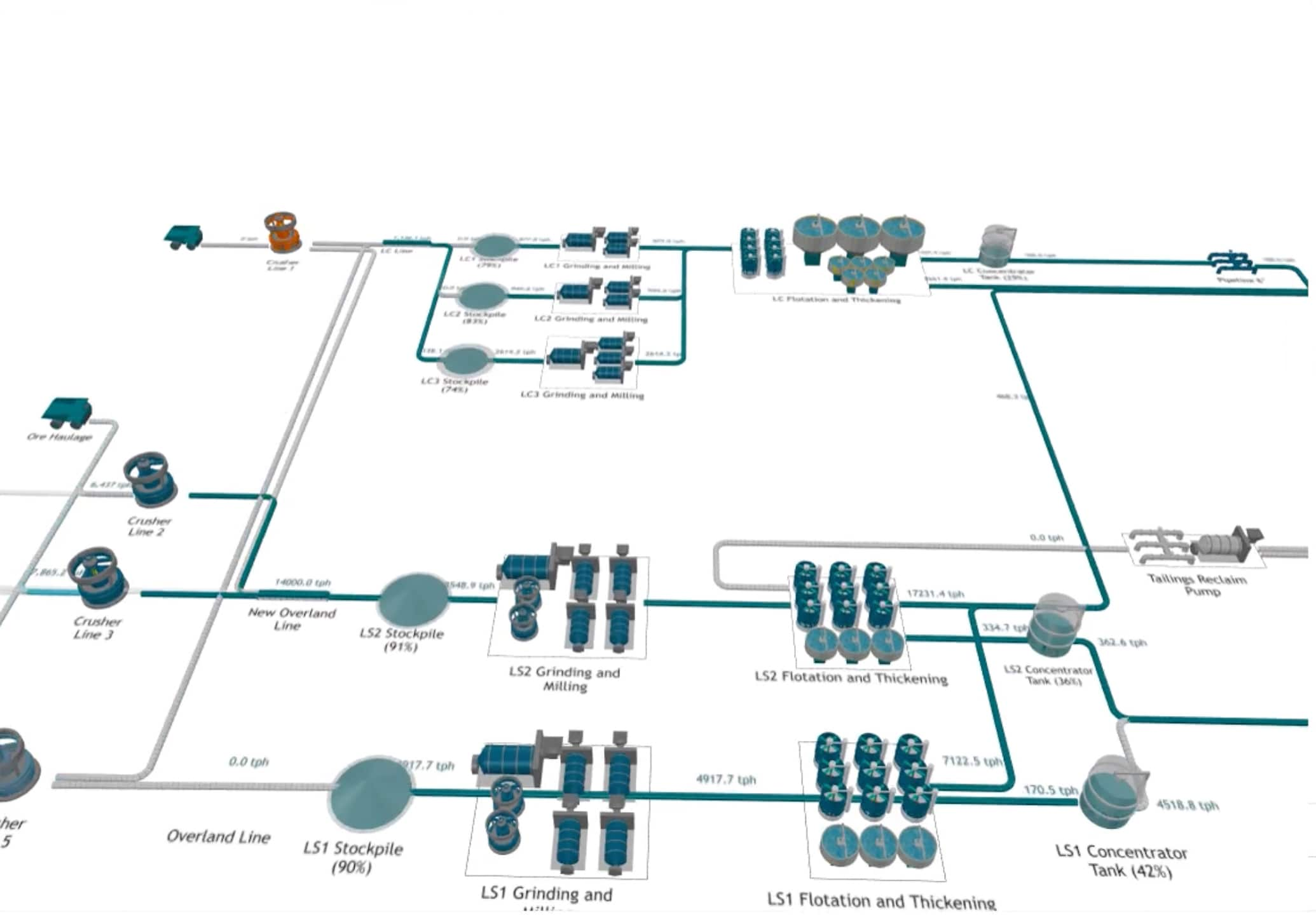
Example of the Escondida Digital Twin Model
Enhancing digital twin insights creation using GenAI
Integrating GenAI with our digital twin models has resulted in faster insights generation, uncovered better opportunities and democratised access, allowing non-technical users to design and perform scenario analysis in natural language for improved business decisions.” – Iván López, Vice President Value Engineering
Our digital twin models have hundreds of input variables and produce thousands of output statistics. Each statistic is captured every minute and leads to millions of data points. How do we design the what-if scenarios? Which input variables should we test? How can we make sense of millions of output data points and compare the multitude of scenarios? Typically, designing the scenarios and extracting insights from the digital twin model requires specialised skills due to the large volumes of data, model complexities and numerous permutations and combinations of scenarios. Integrating GenAI with our digital twin models has resulted in faster insights generation, uncovered better opportunities and democratised access, allowing non-technical users to design and perform scenario analysis in natural language for improved business decisions.
Integrating GenAI with our value chain digital twin models empowers our people to make informed, data-driven decisions, which results in faster and more accurate planning. We are also able to predict the results from unexpected scenarios and prepare for a wider range of potential conditions. By applying knowledge and insight across all the assets we operate, we can optimise performance across the business.
We recently applied GenAI to our digital twin at BMA. Using natural language, the non-technical user can ask a variety of questions. For example, what are the realistic production ranges over the next five years? How does the value chain bottleneck shift and what are the key performance drivers causing the bottleneck? What levers can we pull to increase production? Can you suggest and run new scenarios to increase production? Through GenAI, future performance can be predicted with ease and hidden performance improvement opportunities can be uncovered.
Digital innovation and sustainability practices
A new age of digital innovation is dawning. New tools and technologies can synthesise vast quantities of complex data, better predict future outcomes and improve decision-making. As an industry, we need to find better, more sustainable ways to produce the metals and minerals required for the energy transition. The opportunities to use digital twins and AI to deliver greater efficiency and effectiveness is huge – for example, we can optimise energy and water consumption to reduce greenhouse gas emissions and broader environmental impact. Importantly, it will enable our work environments to be safer and more productive.
Get the latest BHP news alerts, straight to your inbox
Get BHP news first. Stay in touch with our latest updates, investor news and media releases.